Understanding Data Labeling in Machine Learning
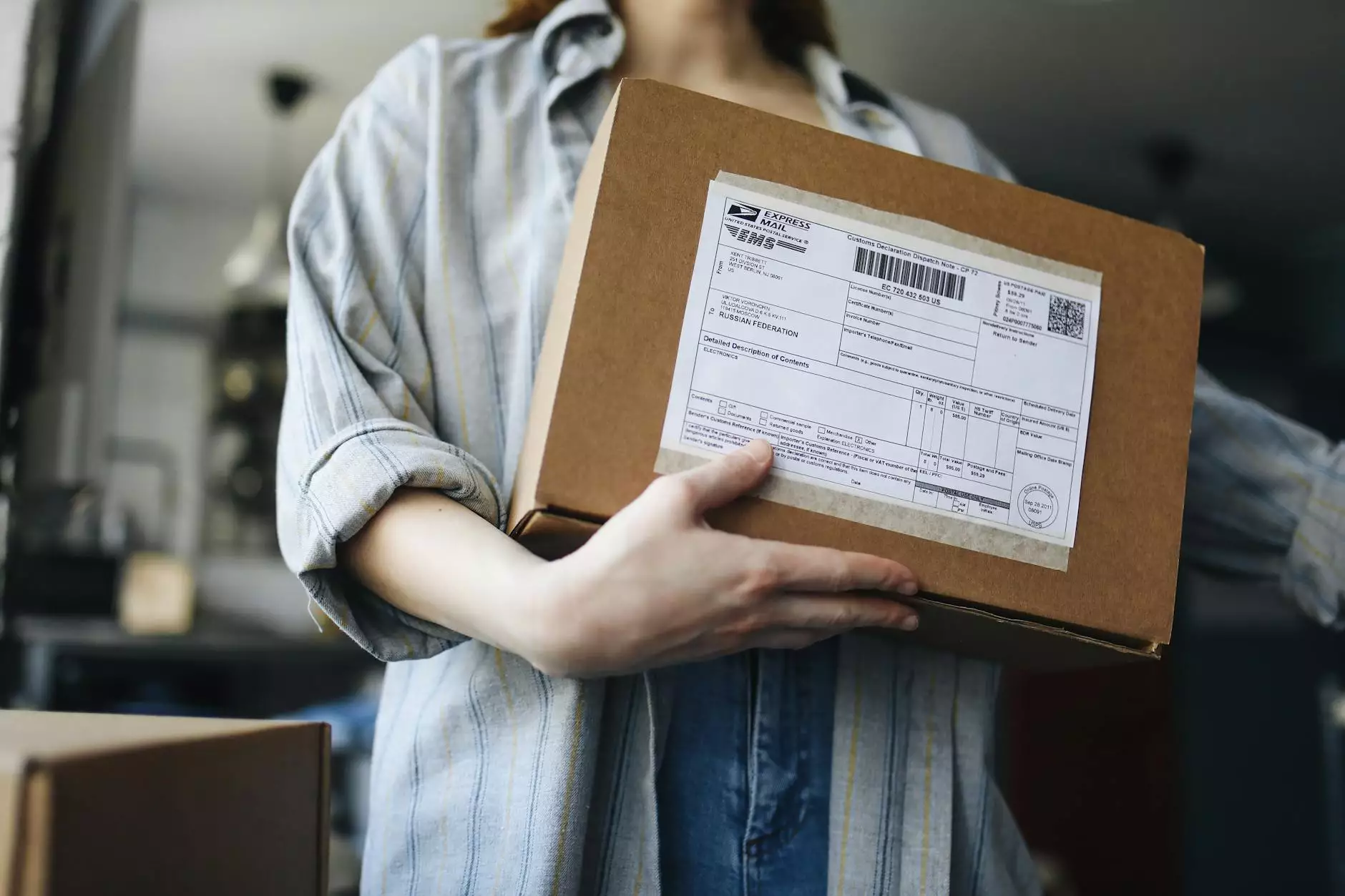
Data labeling is a pivotal process in the realm of machine learning that essentially sets the ground for AI systems to operate effectively. In essence, it involves annotating or tagging data so that machine learning algorithms can understand it and make predictions based on that information. Without high-quality labeled data, the capability of these algorithms to learn, adapt, and make decisions becomes severely compromised.
The Crucial Role of Data Labeling
As businesses increasingly harness the power of artificial intelligence, the need for data labeling has surged. Accurate data sets allow for more informed modeling, leading to better performance from AI systems. Here’s a brief overview of why data labeling is crucial:
- Quality Prediction: In many cases, the effectiveness of a machine learning model is directly linked to the quality of the training data.
- Informed Decision-Making: Businesses use machine learning to drive decisions. Accurate data leads to better insights.
- Efficiency in Training: Well-labeled data reduces the time and resources spent on training models.
Applications of Data Labeling in Various Industries
The applicability of data labeling machine learning spans myriad fields. Here are some of the primary sectors leveraging this process:
Healthcare
The healthcare industry employs data labeling for various applications, including:
- Medical Imaging: Data labeling helps in identifying tumors or anomalies in X-rays and MRIs.
- Patient Records: Leveraging labeled data from historical patient outcomes to predict future health risks.
Autonomous Vehicles
Self-driving technology depends heavily on labeled data:
- Object Recognition: Labeling images and videos enables vehicles to detect pedestrians, cyclists, and road signs.
- Path Planning: Helps in determining optimal routes based on labeled geographical data.
Retail and E-commerce
Retailers utilize data labeling to:
- Customer Behavior Analysis: Segment customer demographics for targeted marketing efforts.
- Inventory Management: Predict stock requirements based on labeled historical sales data.
The Process of Data Labeling
Understanding how data labeling works can provide insights into its significance in machine learning:
Step-by-Step Guide
- Data Collection: The first step involves gathering raw data from various sources.
- Data Preprocessing: Cleaning and organizing the raw data to prepare it for labeling.
- Labeling: Annotators classify and tag the data accurately according to predefined guidelines.
- Quality Assurance: Implementing checks and reviews to ensure labeling accuracy.
- Integration: Finally, the labeled data is fed into machine learning models for training.
Challenges in Data Labeling
Despite its apparent benefits, the data labeling process does come with its challenges:
Quality Control
Ensuring consistent labeling accuracy is crucial; any discrepancies can lead to model inaccuracies.
Scalability
With massive datasets becoming common, scaling the labeling process while maintaining quality can be a significant hurdle.
Cost
Labeled data can be expensive to produce, especially when hiring professionals or using specialized platforms.
Keymakr: Solutions for Enhanced Data Labeling
At keymakr.com, we understand the importance of efficient and accurate data labeling machine learning. We provide advanced solutions tailored for businesses needing high-quality labeled data. Our services cater to various sectors, including home services, keys, and locksmiths.
Benefits of Partnering with Keymakr
- Expertise: Our professionals have deep knowledge of data science and machine learning, ensuring optimal labeling.
- Custom Solutions: We design personalized data labeling strategies tailored to specific business needs.
- Speed: We leverage efficient workflows that drastically reduce the time spent on data labeling.
Future Trends in Data Labeling
The data labeling landscape is continually evolving, influenced by advancements in technology:
Automation and AI-Driven Labeling
Automation tools and AI algorithms are increasingly being used to assist in the labeling process, offering:
- Speed: Automated systems can label data faster than manual methods.
- Cost-Effectiveness: Reducing the need for extensive labor, allowing for scalability.
- Improved Accuracy: With machine learning, systems can learn from previous labeling, reducing human error.
Emphasis on Quality over Quantity
There's a growing recognition that the quality of labeled data is more crucial than the volume. Enhanced methodologies ensure better accuracy, which translates into better performing AI systems.
Conclusion
In conclusion, the role of data labeling in machine learning cannot be overstated. As technology advances and more businesses seek to integrate AI into their operations, the demand for quality data labeling will only increase. By partnering with experts like keymakr.com, businesses in the home services, keys, and locksmiths sectors can ensure they are equipped with the best possible data to fuel their machine learning initiatives.
Call to Action
If your business is looking to optimize its machine learning capabilities through effective data labeling, reach out to keymakr.com today. Let us help you drive success through superior data solutions that enhance your operational effectiveness and decision-making processes.